Rong Gu 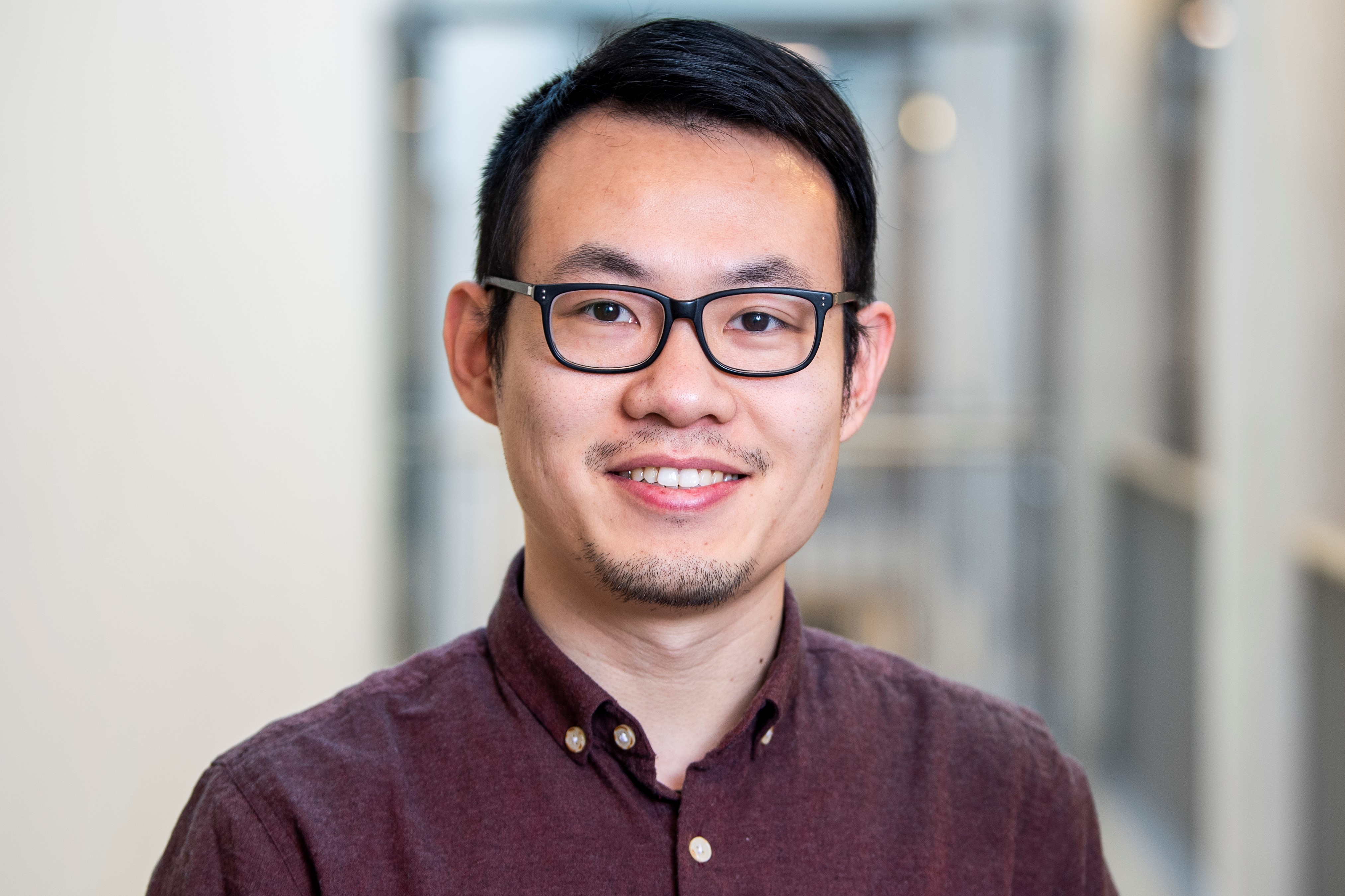
I am a PhD student at Mälardalen University, in the Formal Modeling and Analysis of Embedded Systems group.
I have received a Licentiate in Computer Science from Mälardalen University, Sweden, a Master in Software Engineering from Xi'an Jiaotong Univeristy, China, and a Bachelor degree in Software Engineering from Xi'an Jiaotong University, China. Since 2017 I have become a PhD student in Mälardalen University, under the supervision of Associate Professor Cristina Seceleanu, Professor Kristina Lundqvist, and Senior Lecturer Eduard Enoiu. Post Doc Raluca Marinescu is my former co-supervisor.
More details about me and my work can be found here.
Dissertation Title: Formal Methods for Scalable Synthesis and Verification of Autonomous Systems -
Mission Planning and Collision Avoidance
Dissertation Abstract:
Autonomous systems (a.k.a., agents) are often designed to move and execute tasks, without or with little human intervention. As the agents are often involved in safety- or mission-critical scenarios, ensuring the correctness of mission planning (i.e., path finding and task scheduling) and collision avoidance is crucial for such systems. However, traditional verification approaches, such as testing, are not sufficient to provide such assurance.
Formal methods such as model checking are well known for their rigorous verification based on mathematical models and logic rules, which provide guarantees of the absence of errors in system models. However, employing them entails tackling many challenges such as the complicated formal modeling and the scalability of the algorithmic methods. Additionally, the mission planning concerns the static and predictable factors in the working environment of the agents, such as stationary obstacles and predefined tasks, whereas the collision avoidance focuses on the dynamic and unpredictable factors, such as pedestrians. Consequently, certain questions arise in this context: (i) How can formal methods be applied in providing correctness-guaranteed solutions within a holistic framework that handles both the static mission planning and the dynamic collision avoidance?, and (ii) When the methods for realizing the agents' artificial intelligence, such as machine learning, involve large amounts of data, how to improve the scalability of formal methods when verifying the results of such methods? In this dissertation, we offer answers to the questions by developing solutions in form of new frameworks and algorithms targeting the mentioned problems, implementing the solutions in software tools, and evaluating their performance on real-world applications.
We propose a two-layer framework for formal modeling and verification of agents. The framework separates the discrete mission planning from the continuous movement of agents, which is needed for collision avoidance verification. Additionally, different formal modeling and verification techniques are adopted in the two layers of the framework respectively.
For mission planning, we design two types of tool-supported approaches, one based on graphic search, and one based on learning. The former is sound and complete, and supported by the tools UPPAAL and UPPAAL TIGA. However, the graphic-search approach is not scalable for large numbers of agents. The learning-based solution complements the graphic-search one, by handling more agents, being supported by UPPAAL STRATEGO. As a trade-off, the learning-based method is sound but not complete.
For the verification of collision avoidance, we propose two solutions, the first one based on statistical model checking in UPPAAL SMC, and second one based on the symbolic model checking of UPPAAL STRATEGO. In the second solution, we transform the hybrid agent models, whose verification is undecidable, into a conservative over-approximation as a discrete-time model whose model checking is decidable. These results are proven as theorems in the dissertation.
To support our methods, we develop a toolset named MALTA that enables the automation of model construction and mission planning, and provides a visualization of environment configuration and the resulting mission plans. By using MALTA, we experiment with our novel methods in an industrial use case: an autonomous quarry. The experiment results demonstrate the advantages and weaknesses of different methods used in different types of environments, as well as the applicability of our methods and tool in complex systems.
Papers that are included in the dissertation:
1. Towards a Two-layer Framework for Verifying Autonomous Vehicles
Rong Gu, Raluca Marinescu, Cristina Seceleanu, and Kristina Lundqvist.
Published in 11th NASA Formal Methods Symposium (NFM), Springer, 2019
2. Verifiable Strategy Synthesis for Multiple Autonomous Agents: A Scalable Approach
Rong Gu, Peter G. Jensen, Danny B. Poulsen, Cristina Seceleanu, Eduard Enoiu, and Kristina Lundqvist.
Published the International Journal on Software Tools for Technology Transfer (STTT), Springer, 2022.
3. Synthesis and Verification of Mission Plans for Multiple Agents under Complex Road Conditions
Rong Gu, Eduard Baranov, Afshin Ameri, Eduard Enoiu, Baran Cürüklü, Cristina Seceleanu, Axel Legay, and Kristina Lundqvist.
Submitted to ACM Transactions on Software Engineering and Methodology (TOSEM), ACM, 2022.
4. Probabilistic Mission Planning and Analysis for Multi-agent Systems
Rong Gu, Eduard Enoiu, Cristina Seceleanu, and Kristina Lundqvist.
Published in 9th International Symposium On Leveraging Applications of Formal Methods, Verification and Validation (ISoLA), Springer, 2021
5. Correctness-Guaranteed Strategy Synthesis and Compression for Multi-Agent Autonomous Systems
Rong Gu, Peter G. Jensen, Cristina Seceleanu, Eduard Enoiu, and Kristina Lundqvist.
Submitted to Science of Computer Programming (SCP), Elsevier 2022.
6. Model Checking Collision Avoidance of Nonlinear Autonomous Vehicle Models
Rong Gu, Cristina Seceleanu, Eduard Enoiu, and Kristina Lundqvist.
Published in 24th International Symposium On Formal Methods (FM), Springer, 2021
Papers that are published but not included in the dissertation:
1. Formal Verification of an Autonomous Wheel Loader by Model Checking
Rong Gu, Raluca Marinescu, Cristina Seceleanu, Kristina Lundqvist.
Published in Proceedings of the 6th Conference on Formal Methods in Software Engineering (FormaliSE), ACM, 2018
2. TAMAA: UPPAAL-based Mission Planning for Autonomous Agents
Rong Gu, Eduard Paul Enoiu, and Cristina Seceleanu.
Published in Proceedings of the 35th ACM/SIGAPP Symposium On Applied Computing (SAC), ACM, 2020
3. Verifiable and Scalable Mission-Plan Synthesis for Multiple Autonomous Agents
Rong Gu, Eduard Enoiu, Cristina Seceleanu, and Kristina Lundqvist.
Published in 25th International Conference on Formal Methods for Industrial Critical Systems (FMICS), Springer, 2020. Best-paper Award.
The doctoral dissertation can be downloaded here.